PI: Carole Delenne
Urban water models must be parametrized. Acquiring in situ data in urban water systems remains a costly operation. It is often complexified by accessibility and operational/regulatory constraints. Increasingly available open databases open up a new way to information and data collection.
The research consists in (i) designing data mining and analysis methods suited to the specific objects of the urban environment, (ii) using the producs of geomatics to identify proxy variables that will allow for model parameter estimation, (iii) applying data fusion techniques while accounting for inconsistencies and assessing the propagation of uncertainties within models.
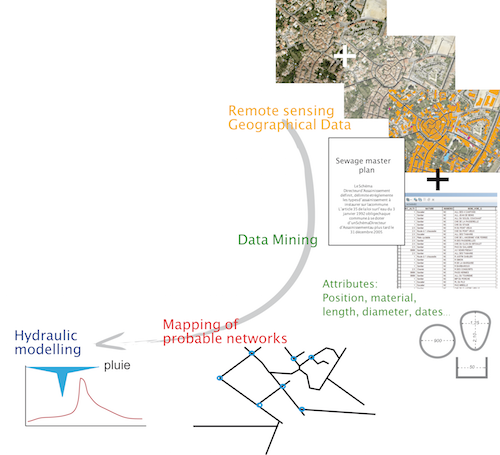
Within the framework of the Cart’Eaux project, a deep learning approach was developed to identify sewer network manhole covers from very high resolution aerial imagery. The Alexnet CNN was trained using a 5 cm resolution image of the Prades-le-Lez city. It was then applied to the Gigean city. The precision and recall were respectively 72 % and 54% (Pasquet et al., 2016 ; Commandré et al., 2017). Several research paths are currently under exploration to improve these results prior to applications over larger areas.
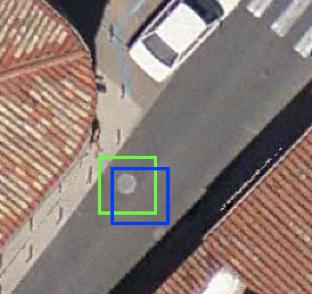
A mapping algorithm in GIS vector format was developed to identify the links between the manhole covers. Objective functions based on rules of practice were used (Chahinian et al., 2019). Remote sensing data uncertainty is accounted for via a stochastic approach, whereby each link in the network is characterized by its probability of occurrence.
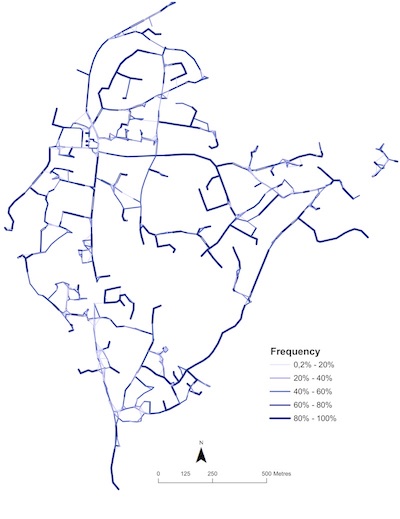
However, the sole knowledge of the network topology is not sufficient for hydraulic modelling. The characteristics of the nodes and conduits in the model must be fully known via specific, complete and consistent attribute tables.

Within the MeDo project, texts harvested automatically from the WWW are classified (relevant/not relevant) using an artificial neural network. The relevant documents are annotated for thematic, spatial and temporal entities using a Named Entity Recognition module trained on a test corpus. The detected entities are linked together, thus allowing for display within a GIS.
n Y. Belghaddar’s PhD thesis, Graph Neural Networks are used for Missing Value Imputation (MVI). A meta-model has been implemented within the Moose platform, so that each piece of data has the same format regardless its source and original format. This meta-model is the first step in data fusion.